
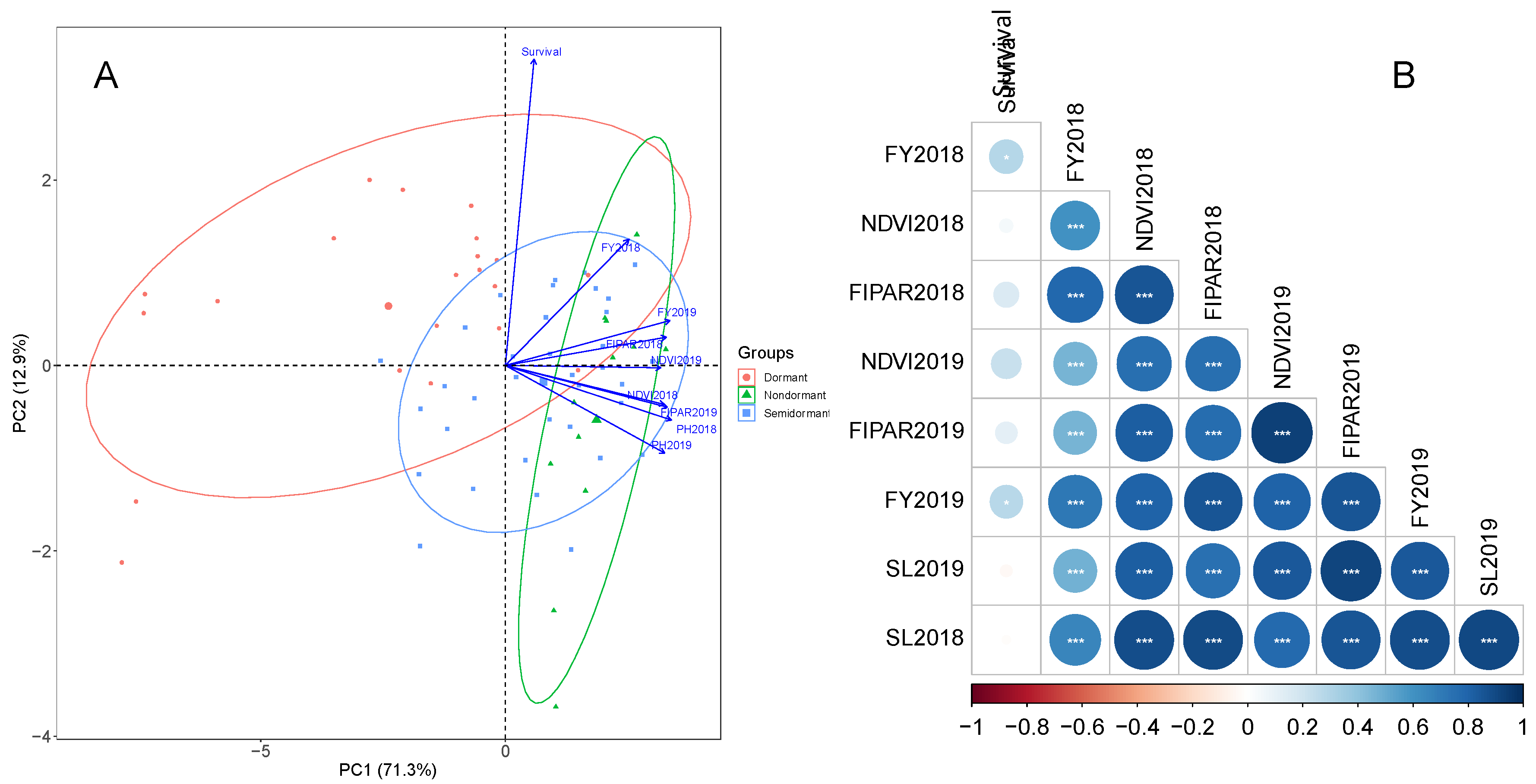
Once the design and treatment structure are recognized the correct analysis follows easily. Linear mixed models (LMM) are particularly useful in dealing with the multi-stratum structure of an experiment, as they will recognize these layers and identify at what stratum there is pseudo-replication or true replication. Here, these leaves will also be MU, but in this case, they correspond to a subsampling within each plant.Ĭareful identification of the design structure of an experiment, or equivalently of the arrangement (or layers) of the observations will allow us to identify the existence of pseudo-replication, and also it will help us to correctly specify this multi-stratum structure into a statistical model. For example, in our experiment we could have counted insects in a sample of two leaves per plant.

You can identify similar cases, for example where we obtain several measurements from a single EU, or even several measurements from a given MU. The difficulty comes when we take measurements ( e.g., plant height) from each of the plants, and therefore the EU differs from the MU. Treatment levels should always be applied randomly to different EU, as in the above example where a treatment level ( e.g., fertilizer) was applied to a plot, and thus all four plants within the plot have the same treatment level. However, the most important aspect is to clearly distinguish between experimental units (EU) and the measurement units (MU). Pseudo-replication is not always easy to recognize, particularly for experiments that contain multiple layers or strata. We will discuss both aspects with emphasis on the use of linear models (LM) and linear mixed models (LMM).
PLOT ASREML R TYPE HOW TO
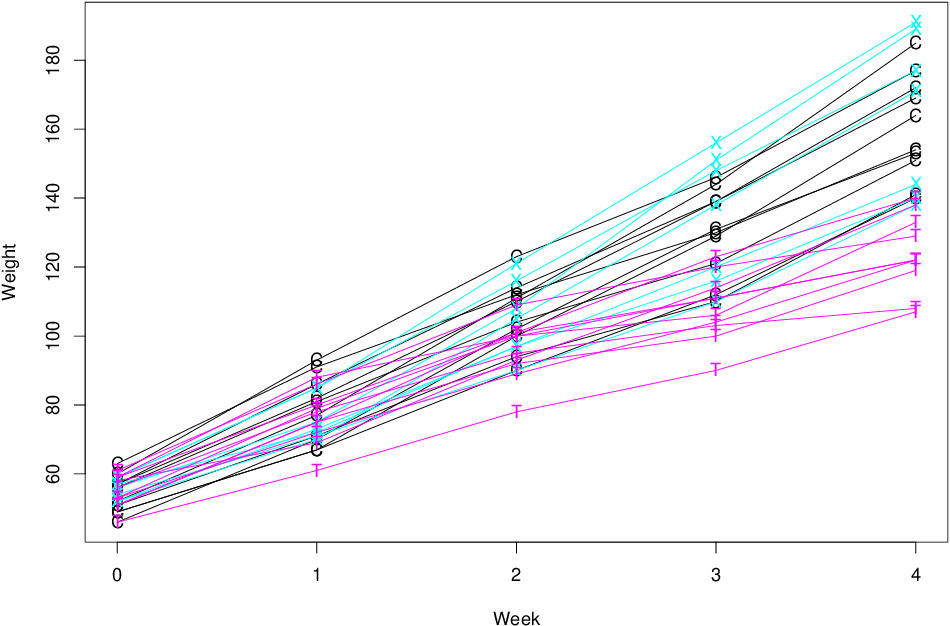
In this experiment one treatment ( e.g., control) is applied to four plots at random, and the other treatment ( e.g., fertilizer) to the remaining four plots. To illustrate this, we will consider an experiment that compares two treatments applied to eight plots, each containing four plants (see figure below). Almost always this incorrect identification will make the statistical analyses invalid. This can occur at the planning or execution stage of a study, or during the statistical analyses. Pseudo-replication is associated with the incorrect identification of the number of samples or replicates in a study.
